Artificial intelligence is rapidly transforming industries, offering businesses and individuals new ways to solve problems, streamline tasks, and enhance decision-making.
However, AI is not perfect, and it still requires improvements to reach its full potential.
By implementing these strategies, you can enhance the efficiency and capabilities of your AI models, making them more effective and reliable.
If you’re looking to enhance your AI applications, you are at the right place!
We have mentioned 10 practical ideas you can easily implement.
Action 1. Collect High-Quality Data
The foundation of any AI application is the data it is trained on. High-quality data, much like an Intelligent Laptop that optimizes performance, and ensures accurate predictions and efficient learning. Poor data can lead to incorrect results, so focusing on clean and relevant datasets is essential for AI success.
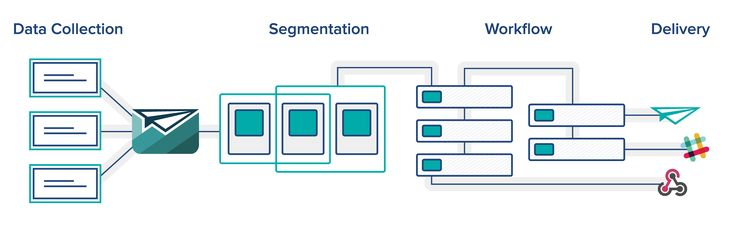
Key tips for collecting quality data:
- Ensure data diversity to cover various use cases.
- Remove or fix any data errors or inconsistencies.
- Regularly update your dataset to keep it fresh and relevant.
Action 2. Regular Model Updates
AI models need regular updates to stay relevant and perform at their best. The environment in which AI operates often changes, and new data or trends can affect the model’s accuracy.
Therefore, continuously refining and training your models with fresh data is essential for improving their performance over time.
Here are five actionable steps for regular model updates:
- Retrain models periodically: New data should be used to retrain models every few months.
- Adjust for bias: Ensure that the model is not skewed toward a specific group or pattern.
- Test performance regularly: Run the model through tests to evaluate how well it performs in different situations.
- Incorporate feedback: Gather feedback from users to refine the model based on their experiences.
- Monitor AI drift: AI drift happens when the model starts to underperform due to changing data trends.
Action 3. Implement Ethical AI Practices
Ethical concerns in AI are growing, especially regarding data privacy and fairness. To improve your AI application, it is essential to implement ethical practices at every step.
Ethical AI ensures that your models make fair, transparent, and unbiased decisions, while also respecting user privacy.
Best practices for ethical AI:
- Prioritize data privacy by securing sensitive user information. A 2024 Cisco study revealed that 27% of organizations have banned the use of Generative AI tools due to data privacy and security concerns.
- Make your algorithms transparent, explaining how decisions are made.
- Test for biases in your AI systems to ensure fairness for all users.
Why does ethics matter in AI?
Ethical AI practices help prevent legal issues and build trust with users, encouraging them to adopt your AI systems. Similarly, just as an intelligent laptop adapts to user needs, an ethical AI model fosters long-term acceptance and success in the market.
Action 4. Improve Model Explainability
One of the biggest challenges with AI is its “black-box” nature. Often, AI systems provide decisions without explaining how they reached them. This lack of transparency can make it hard to trust AI applications. Improving model Explainability means ensuring that users can understand how an AI system arrived at a particular decision.
The key to improving explainability is developing AI systems that are interpretable by humans. You can achieve this by using simpler algorithms or by creating detailed reports and visualizations that show how inputs lead to specific outcomes. Providing explanations increases the trust in your AI models and helps users better understand their behaviors.
Action 5. Optimize Performance Through Hyperparameter Tuning
Hyperparameters are settings in an AI model that affect its performance but are not directly learned during training. These parameters significantly influence the model’s ability to make accurate predictions. Tuning these hyperparameters is essential for enhancing AI applications. It involves adjusting settings like learning rates, the number of hidden layers, and batch sizes to ensure the model is performing optimally.
Here are five strategies to optimize performance through hyperparameter tuning:
- Grid search: Try different combinations of hyperparameters to find the best settings.
- Random search: Randomly sample hyperparameter values to quickly find a good combination.
- Bayesian optimization: Use statistical methods to fine-tune hyperparameters efficiently.
- Early stopping: Stop training when performance stops improving to prevent overfitting.
- Cross-validation: Use cross-validation techniques to ensure the model performs well on unseen data.
Action 6. Address AI Bias And Fairness
AI models can inadvertently develop biases due to biased data or flawed algorithms. These biases can result in unfair or inaccurate predictions, which can harm certain user groups. By addressing AI bias, you can make your application more inclusive and equitable. It’s crucial to regularly assess the data and algorithms to ensure fairness in all AI applications.
How to address bias in AI:
- Regularly audit your models for biases and adjust them accordingly.
- Use diverse data sources that represent different demographics and experiences.
- Implement fairness-aware algorithms that prevent biased decision-making.
Why is fairness important?
Fairness in AI is important because it ensures that all individuals are treated equally, no matter their background or characteristics. Ensuring that AI applications are fair and unbiased will make them more effective and socially responsible.
Action 7. Improve Real-Time Learning
Real-time learning is essential for AI applications that require immediate decision-making. It involves continuously feeding new data into the system so that the model can adapt quickly to changes.
For instance, an intelligent laptop can enhance real-time learning by processing data swiftly, making it crucial for applications like autonomous driving or fraud detection. By focusing on real-time data processing, you can improve the responsiveness and accuracy of your AI systems.
Key benefits of real-time learning:
- Improved decision-making in dynamic environments.
- Reduced delays between data collection and action.
- Enhanced user experience with quicker responses.
Action 8. Enhance User Interface (UI) And User Experience (UX)
A great user interface (UI) and user experience (UX) are essential to the success of AI applications. No matter how powerful your AI model is, if users find it difficult to interact with, they won’t adopt it. Improving the UI and UX involves simplifying the interface, ensuring it is easy to navigate, and creating a seamless experience for users.
Key tips to enhance UI/UX:
- Simplify complex tasks and workflows to improve accessibility.
- Use clear, concise language to guide users through the application.
- Offering timely feedback and support enhances user satisfaction and engagement.
Incorporating AI into UX design is expected to cut down design time by 30% through automation by 2026
Action 9. Scale Your AI Solutions
Scalability is crucial when growing AI applications to handle more users, data, or tasks. As your AI model performs well, you may face increased demand, which requires scaling the system. By ensuring that your AI application is designed for scalability, you can handle growth without compromising performance.
How to scale AI solutions:
- Use cloud-based services to support increased data storage and processing needs.
- Optimize algorithms to ensure they can handle large datasets efficiently.
- Invest in scalable infrastructure to support growth without performance loss.
Action 10. Foster Cross-Industry Collaboration
AI is a rapidly evolving field, and collaboration across industries can foster innovation. By working with experts from various sectors, you can discover new applications, share best practices, and gain insights that help improve your AI applications. Cross-industry collaboration also helps you stay updated on the latest trends, allowing your AI models to adapt quickly to changes in the market.
Benefits of collaboration:
- Access to diverse knowledge and expertise.
- Faster innovation and problem-solving.
- Opportunities for integrating AI in various fields.
Conclusion
Improving AI applications is an ongoing process that requires focus on data quality, regular updates, ethical practices, and performance optimization. If you’re working on a smart AI laptop, applying these 10 actionable ideas will make your AI applications more effective, transparent, and reliable. Whether refining existing models or developing new ones, these strategies enhance the performance and impact of your AI solutions, driving innovation.